Getting Started with Responsible AI: A Look at How Leading Companies Are Trying to Make It Work
- Jessica Hall
- Aug 24, 2024
- 6 min read
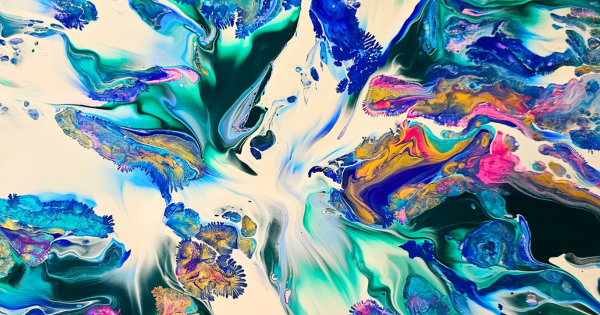
There is a lot of excitement about the opportunity AI offers to make things easier and better and a lot of concerns about what can go wrong. We’ve learned from the rise of social media to be skeptical and looking out for potential challenges. Thankfully there are a lot of smart people in government, academia, nonprofits and industry working to create frameworks and tools to manage this.
“AI systems are the product of many different decisions made by those who develop and deploy them. From system purpose to how people interact with AI systems, we need to proactively guide these decisions toward more beneficial and equitable outcomes,” according to Microsoft.
Previously, I explored how the big players are driving AI transformation. Now I’m looking into how the big players are approaching Responsible AI. IBM encourages organizations to create their own principles based on their industry and client needs, with continuous education and diversity of input. Google, McKinsey, and Accenture focus on a human centered approach and principles. The Algorithmic Justice League advocates for equitable AI, meaningful transparency, and continuous oversight.
There’s a lot to consider here. This guide is a starting point—a framework to help us explore the terrain of responsible AI. We don’t need all the answers right now, but we do need to start somewhere. By staying flexible and open to learning, we can adapt as new standards and regulations come into play. Let’s dive in and navigate this together.
What are the key elements of Responsible AI?
Fairness means ensuring that artificial intelligence systems don't discriminate against or unfairly impact certain individuals or groups. The goal is to create AI that's equitable and inclusive, steering clear of harmful biases. Here are some examples of how companies do this:
Fairness
IBM: Advocates for diverse and representative data, bias-aware algorithms, and diverse development teams. They recommend using their AI Fairness 360 toolkit to examine and mitigate discrimination and bias.
Google: Stresses the importance of using representative datasets, checking for unfair biases, and designing models with concrete goals for fairness and inclusion.
Microsoft: Engaged a sociolinguist to improve speech-to-text technology and address performance gaps, focusing on fairness and inclusivity.
McKinsey: Emphasizes human-centric AI with oversight to mitigate unfair discrimination and bias.
Accenture: Highlights fairness as a core principle in their AI framework, supported by tools like the AI Fairness Toolkit.
Transparency & Explainability
AI systems are increasingly making decisions that affect our daily lives - from loan approvals to job applications and even healthcare diagnoses. When we talk about transparency and explainability in AI, we're not just aiming for technical clarity. We're focused on making these systems understandable to you, the person on the receiving end of AI-driven decisions.
It's not enough for AI to be a "black box" that spits out results. The goal is to create AI systems that can explain their decisions in ways that make sense to the people affected by them. And here's the key: the success of this explanation should be judged by you, not the AI developers. If you can't understand why an AI made a particular choice about your life, then the system isn't truly transparent or explainable. How are companies tackling this challenge?
IBM: Focuses on explainability through prediction accuracy, traceability, and decision understanding.
Cisco: Prioritizes transparency in AI involvement in decision-making and provides choices in technology use.
Google: Recommends building in disclosures and understanding the inferences of datasets and models.
McKinsey: Advocates for accountable and transparent AI systems with clear oversight across the lifecycle.
Deloitte: Calls for transparent and explainable AI systems to ensure participants understand how decisions are made.
Accountability
In the world of AI, accountability is about creating a clear chain of responsibility for AI systems that are increasingly making important decisions in our lives. From social media algorithms to automated hiring processes, AI is everywhere - but who's in charge when these systems make mistakes or cause harm?
Accountability in AI means establishing clear lines of responsibility, from the developers who create the systems to the companies that deploy them. It's about ensuring that there are real consequences for AI-related failures and a commitment to fixing issues when they occur. Most importantly, it's about protecting you, the user or subject of AI decisions, by ensuring there's always a human or organization that can be held responsible.
How are companies approaching this challenge?
Cisco: Ensures accountability through executive oversight, controls, incident management, and external engagement.
Google: Recommends a governance structure with focal points and an ethics board to support compliance.
Accenture: Emphasizes the importance of accountability throughout the AI lifecycle, with a focus on trust.
McKinsey: Highlights the need for responsible and accountable AI systems, ensuring clear ownership of outcomes.
Deloitte: Stresses the importance of responsible AI that includes clear policies for accountability.
Robustness & Reliability
As AI systems take on more critical roles in our lives - from diagnosing diseases to driving cars - we need to ensure that these systems work consistently and correctly. This includes consistently performing, preventing attacks, preventing model drift and analyzing failures. How are companies working to make AI more dependable?
IBM: Encourages robust and reliable AI systems, focusing on governance practices and integration across the lifecycle.
Cisco: Includes reliability as a core principle in their AI framework, ensuring systems are dependable.
Google: Advocates for continuous testing, monitoring, and updates to ensure system robustness.
McKinsey: Prioritizes the development of AI systems that are robust and reliable, meeting industry-leading standards.
Deloitte: Calls for robust and reliable AI to reduce potential risks and ensure consistent performance.
Privacy & Security
Protecting data, preventing attacks, managing access controls, running audits and managing compliance all needed to be considered throughout the entire lifecycle. How are companies working to protect data and secure systems?
IBM: Incorporates privacy as a key aspect, ensuring data protection throughout the AI lifecycle.
Cisco: Focuses on privacy and security in their AI principles, with controls baked into design processes.
Google: Recommends monitoring systems after deployment to maintain privacy and security.
McKinsey: Develops AI systems with a focus on privacy, security, and confidentiality.
Deloitte: Emphasizes the importance of AI systems that respect privacy and are secure against threats.
Continuous Improvement & Oversight
In any technology effort, we need measure and continually improve our skills, practices and performance. In Responsible AI this means we need to invest in monitoring, measuring towards KPIs and Key Risk Indicators (KRIs).
IBM: Promotes continuous improvement through ongoing training and governance practices.
Cisco: Uses incident management and industry leadership to maintain oversight and drive improvements.
Google: Stresses the need for continuous testing, monitoring, and updates post-deployment.
McKinsey: Establishes standards for continuous learning, adapting AI systems to align with ethical and legal standards.
Accenture: Emphasizes continuous oversight and the need for adaptable frameworks to manage AI risks effectively.
Honesty & Respect
At the heart of any effort focused on responsibility needs to have honesty and respect at the core. No one expects perfect but we do expect that people will acknowledge their mistakes and repair them
Microsoft: Demonstrates honesty by acknowledging and addressing past mistakes in AI performance and improving through expert engagement.
The Algorithmic Justice Leagues focuses on raising awareness about AI impacts and building a movement for equitable and accountable AI, here is a recent interview with Dr. Joy Buolamwini.
Accenture: Highlights the importance of honesty and trust in their responsible AI framework, with a focus on transparency and respect for user data.
How do they implement it?
Ethical AI Frameworks and Principles:
Microsoft’s Responsible AI Standard providing actionable guidance.
Cisco’s AI framework focusing on transparency, fairness, accountability, privacy, and security.
McKinsey’s Trustworthy AI framework outlining principles like reliability, transparency, fairness, safety, and ongoing monitoring.
IBM: Explainability, fairness, robustness, transparency, and privacy.
Governance and Oversight Structures:
Companies have implemented AI ethics boards, training, and advocacy networks to guide ethical decisions.
Creating incident management practices and controls integrated into AI design.
Customizable Frameworks and Toolkits:
PwC and Accenture offering customizable frameworks and tools for AI governance and ethical implementation.
Google’s practices and tools like the "What If Tool" for monitoring and assessing AI fairness and biases.
AI Fairness 360 Toolkit (IBM): Open-source toolkit to examine and mitigate discrimination and bias in machine learning models.
Image: A recent fluid art painting that I was super happy with